By John Blyler, Contributing Editor
At the beginning of his keynote at DAC 2021, Professor Kurt Keutzer from the graduate school in EECS at UC-Berkeley promised to clarify three very popular but misunderstood terms and their interrelationship with electronic design automation (EDA): Artificial Intelligence(AI), Machine Learning (ML), and Deep Learning (DL).
“Given the amount of recent press devoted to these areas, it’s amazing that these terms are rarely clarified, and they are often used synonymously,” said Keutzer during his interview before DAC2021 with Semiconductor Digest. He felt that was a big mistake as each of these areas has its own domains of expertise, and to be appreciated, they must be clearly differentiated.
“Look at the table of contents of the standard textbook on the topic of AI by my Berkeley colleague Stuart Russell, entitled, naturally, Artificial Intelligence. It could be the table of contents of a book on Electronic Design Automation (EDA),” observed Keutzer. Sure enough, AI relies on many familiar techniques that are widely used in EDA: search, backtracking, propositional logic, first-order logic, knowledge representation, probabilistic reasoning, and so forth.
Since its inception, EDA has been employing these traditional AI techniques, and the two fields have co-evolved together, said Keutzer. Furthermore, in many areas, such as propositional reasoning, search, and model-checking, EDA researchers have performed the most advanced research in the field.
“I honestly believe that we should have just as many EDA researchers giving keynote addresses at AI conferences such as NeurIPS as we have AI notables giving keynotes at DAC and other EDA conferences,” explained Keutzer.
Machine Learning
Moving on to machine learning, Keutzer cited another Berkeley colleague, Michael Jordan, who viewed machine learning as a sub-discipline of statistics. This was a bit surprising, as Jordan is widely acknowledged as one of the principal researchers in ML development. When Keutzer pressed Jordan for a definition of the area, Jordan suggested “algorithms and supporting theory for making predictions and decisions under uncertainty based on observed data.”
“To my knowledge, machine learning algorithms are not as ubiquitous in EDA as deterministic traditional AI algorithms and are most commonly applied wherever we have to cope with manufacturing variation,” said Keutzer. Nevertheless, he expected the industry to see them more broadly applied as both EDA vendors and their customers began to harvest the latent value in their design data.
The taxonomy was rounded off with Deep Learning. Here Keutzer avoided a formal definition and noted that in its current application, Deep Learning is focused on the design and training of Neural Nets, a particular family of algorithms in the machine learning toolbox. What’s astonishing is that this one family of machine learning algorithms is currently providing the best solution to a wide variety of problems in computer vision, speech recognition, natural language understanding, and recommendation systems.
“I’ve never seen an era of computer science in which one algorithmic approach was so successful across such a broad range of applications,” exclaimed Keutzer. How is this going to impact EDA?
Keutzer left that answer to the other keynote speakers at DAC, namely Jeff Dean and Bill Dally. Their presentations talked about specific ways that Deep Learning algorithms can be applied to traditional EDA problems. Instead, Keutzer focused on the exciting new business opportunities Deep Learning offers the EDA industry.
“Deep Learning, embedded in edge, mobile, and IoT devices, is going to bring greater intelligence to every aspect of our lives, and I believe the EDA industry can play a significant role in making that all happen, said Keutzer. “But to learn how, you’ll have to come to my talk on Thursday!” Or see the on-demand version provided to registered attendees during the week following DAC.
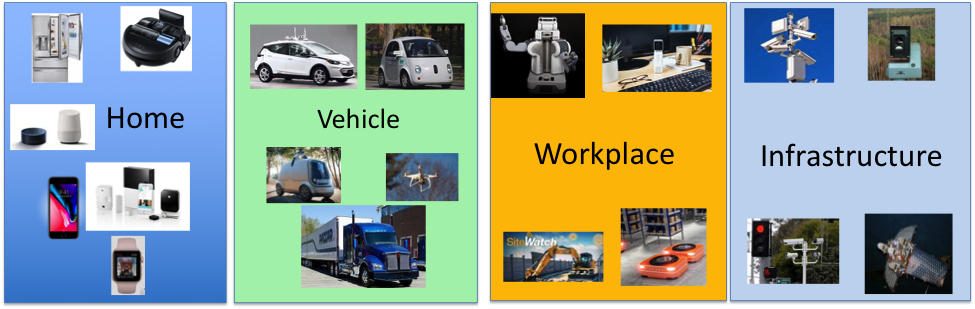